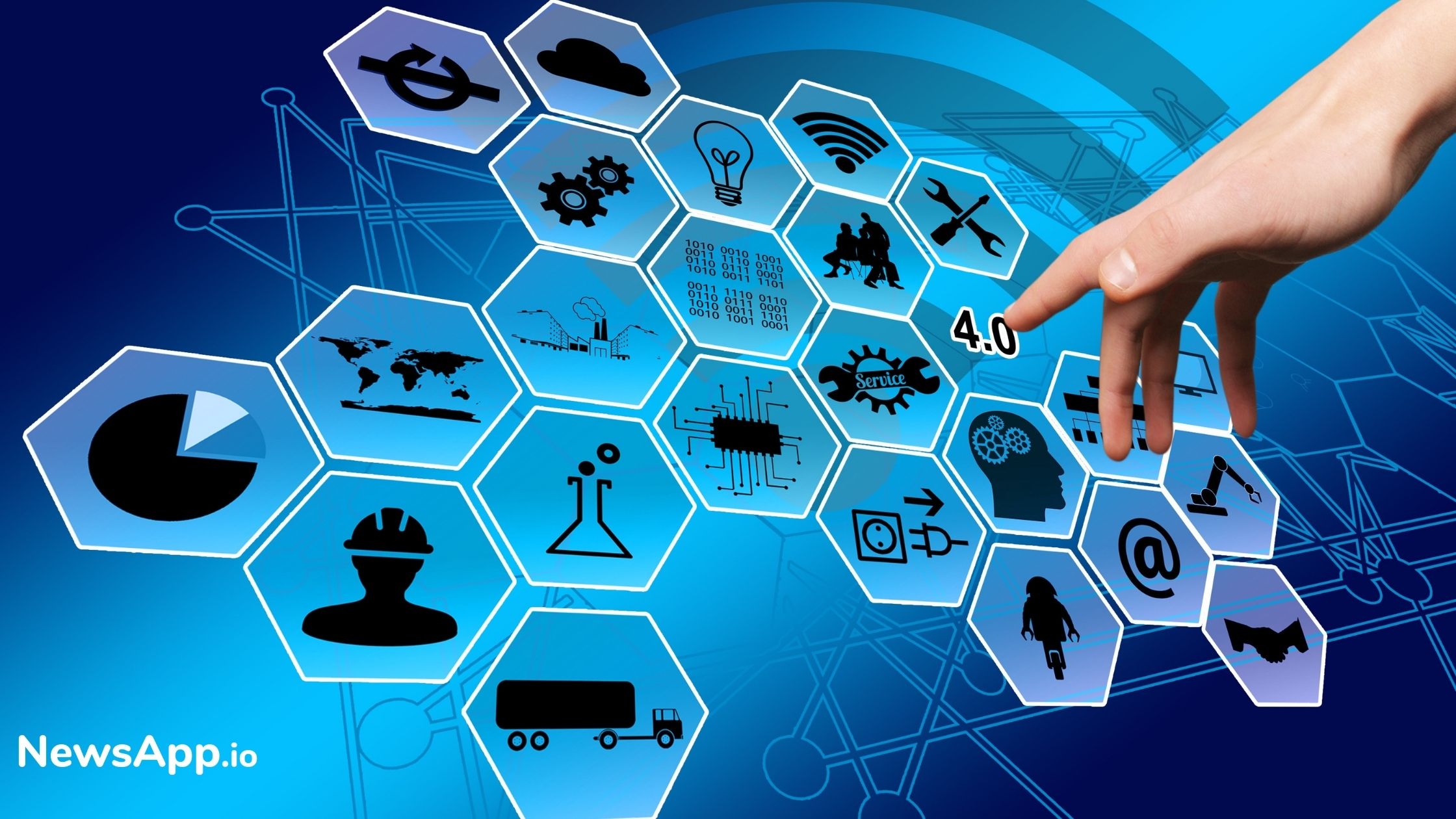
- Sep 19, 2018
5 Challenges faced by Machine Learning
Although the future seems to be promising with technologies like Artificial Intelligence and Machine Learning, it still has some Limitations. Following are 5 challenges faced by ML:
1. Reasoning Power
This is one area where ML has not mastered successfully. Machine Learning lacks the ability to think as to why a certain method is happening that way or introspect their own outcomes. It lacks the ability to think beyond their intended application2. Contextual Limitation
ML has the ability to learn letters, words, sentences and even the syntax. However, where they fail is in the area of the context of the language. It is said that algorithms fail to understand the context of the language.3. Scalability
It can be seen that data is growing at an enormous rate and is said to have many forms which are said to largely affect the scalability of an ML project. When it comes to algorithms, they need to be updated constantly in order to adapt to new changes in order to handle the data.4. Regulatory Restriction for Data in ML
For ML to function, it requires a massive amount of data. Data includes both private as well as general information. The problem arises since most tech companies have privatised their data and this data is required by ML applications. Though data is anonymised at times it still has the ability to become vulnerable. Hence regulatory rules are imposed heavily.5. Internal Working of Deep Learning
Deep Learning is said to power applications which includes voice recognition, image recognition and many more through artificial neural networks. It is said that advanced DL still baffles researchers in terms of its working and efficiency. Since the internal agenda is not known deep learning is said to be dubbed as a 'black box'.Read more at analyticsindiamag.com